Crowding consists of large capital pools chasing related strategies. Within the hedge fund industry, long equity portfolios crowd into several clusters with similar systematic (factor) and idiosyncratic (residual) bets. This hedge fund clustering is the internal structure of crowding. We illustrate the large-scale hedge fund clustering and crowded bets within the largest cluster. Allocators and fund followers without a handle on this phenomenon may be investing in an undifferentiated portfolio prone to liquidation, or paying high active fees for consensus factor exposures.
Hedge Fund Crowding and Hedge Fund Clustering
Our articles on hedge fund crowding analyze the factor (systematic) and residual (idiosyncratic) exposures of HF Aggregate, which consists of the long equity holdings of all U.S. hedge fund portfolios tractable from regulatory filings. Most analyses of crowding overlook bets shared by fund groups within the aggregate. To explore this internal structure of hedge fun crowding, in 2014 AlphaBetaWorks pioneered research on hedge fund clustering. Here we update this analysis with Q4 2015 holdings data.
Hedge Fund Clusters
Note that simplistic analysis of holdings overlap fails to measure fund similarity. Since their variance is overwhelmingly systematic, two funds with no overlapping positions but similar factor exposures can track each other closely. To identify clusters of funds without these deficiencies, we analyze factor and residual exposures of every portfolio relative to every other portfolio using the AlphaBetaWorks’ Statistical Equity Risk Model, a proven tool for forecasting portfolio risk and future performance. For each portfolio pair we estimate the future relative volatility (tracking error). The lower the expected relative tracking error between two funds, the more similar they are to each other.
Once each hedge fund pair is analyzed – hundreds of thousands of factor-based risk analyses – we find funds with similar exposures and build clusters (related to phylogenic trees, or family trees) of funds. We use agglomerative hierarchical clustering with estimated future relative tracking error as the metric of differentiation or dissimilarity. The resulting clusters capture similarities of all analyzable U.S. hedge fund long equity portfolios:
The largest cluster contains approximately 40 funds. It and other large clusters warrant careful scrutiny by allocators: those invested in a portfolio of clustered funds may be paying high active fees for a handful of consensus factor and stock-specific bets.
The AQR-Adage Hedge Fund Cluster
The AQR-Adage Cluster, named after two of its large and similar members, has recently been the largest cluster of hedge funds’ long equity portfolios:
A flat diagram illustrates the distances (estimated future tracking errors) between its members:
This cluster’s aggregate portfolio is similar to the U.S. equity market. We estimate only 1.8% tracking error of the AQR-Adage Cluster relative to the Russell 3000 Index.
Source | Volatility (ann. %) | Share of Variance (%) |
Factor | 1.26 | 48.23 |
Residual | 1.31 | 51.77 |
Total | 1.82 | 100.00 |
Put differently, we expect this cluster’s aggregate annual long portfolio return to differ from the market by more than 1.8% only about a third of the time.
AQR-Adage Cluster’s Factor (Systematic) Crowding
Below are this cluster’s significant factor exposures (in red) relative to the Russell 3000’s exposures (in gray):
Market (high-beta) and Size (small-cap) are the primary sources of the relative factor risk:
Factor | Relative Exposure | Factor Volatility | Share of Relative Factor Variance | Share of Relative Total Variance |
Market | 5.31 | 12.46 | 38.20 | 18.42 |
Size | -8.23 | 8.09 | 22.14 | 10.68 |
Oil Price | 1.42 | 29.43 | 18.84 | 9.09 |
Value | -3.90 | 12.91 | 11.29 | 5.44 |
Finance | -6.56 | 5.08 | 11.05 | 5.33 |
Utilities | -2.21 | 11.28 | 6.05 | 2.92 |
Communications | -1.18 | 11.98 | 2.79 | 1.35 |
Health | -1.57 | 7.22 | -2.97 | -1.43 |
FX | 1.50 | 7.28 | -3.29 | -1.59 |
Energy | -2.07 | 11.77 | -4.56 | -2.20 |
(Relative exposures and relative variance contribution. All values are in %. Volatility is annualized.)
AQR-Adage Cluster’s Factor Crowding Stress Tests
AQR-Adage Cluster’s Maximum Outperformance
Given the AQR-Adage Cluster’s macroeconomic positioning (Long Market, Short Finance, Value, and Size), it would experience its highest outperformance in an environment similar to the 1999-2000 dot-com boom:
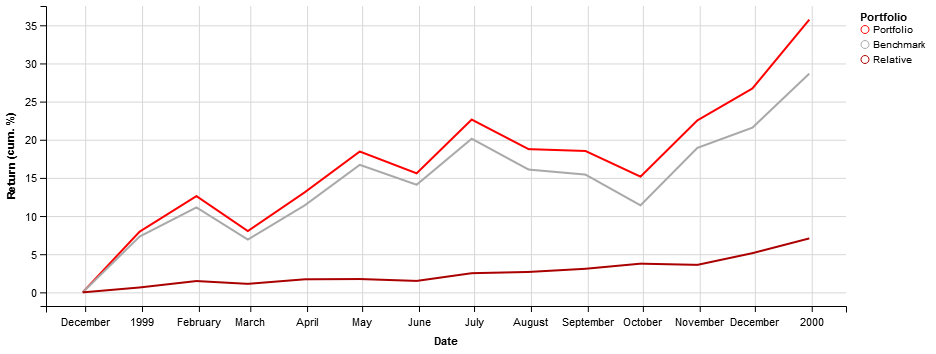
Historical Scenario that Would Generate the Highest Relative Performance for the AQR-Adage Hedge Fund Cluster in Q4 2015
Factor | Return | Portfolio Exposure | Benchmark Exposure | Relative Exposure | Portfolio Return | Benchmark Return | Relative Return |
Market | 31.52 | 107.31 | 102.00 | 5.31 | 34.06 | 32.21 | 1.85 |
Finance | -19.62 | 12.76 | 19.32 | -6.56 | -2.62 | -3.95 | 1.33 |
Oil Price | 128.16 | 0.42 | -1.00 | 1.42 | 0.39 | -0.93 | 1.32 |
Size | -10.49 | -9.11 | -0.88 | -8.23 | 0.96 | 0.09 | 0.87 |
Value | -21.96 | -4.05 | -0.15 | -3.90 | 0.90 | 0.03 | 0.87 |
AQR-Adage Cluster’s Maximum Underperformance
These exposures would deliver the AQR-Adage Cluster its highest underperformance in an environment similar to the 2000-2001 .com crash:
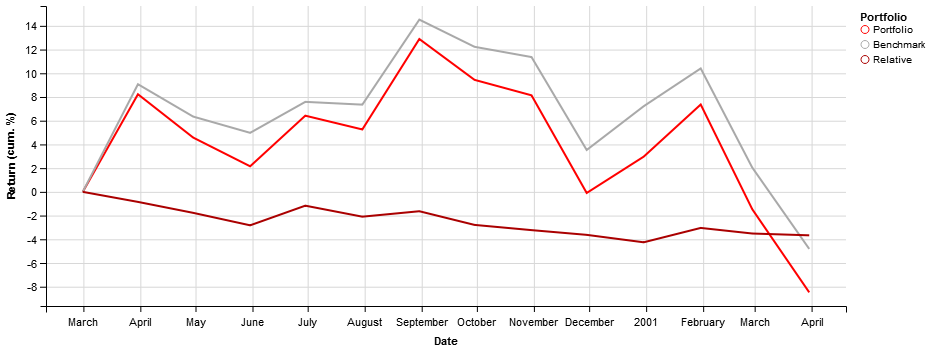
Historical Scenario that Would Generate the Lowest Relative Performance for the AQR-Adage Hedge Fund Cluster in Q4 2015
Factor | Return | Portfolio Exposure | Benchmark Exposure | Relative Exposure | Portfolio Return | Benchmark Return | Relative Return |
Finance | 47.97 | 12.76 | 19.32 | -6.56 | 5.39 | 8.24 | -2.85 |
Value | 86.46 | -4.05 | -0.15 | -3.90 | -2.67 | -0.10 | -2.57 |
Utilities | 52.32 | 0.98 | 3.19 | -2.21 | 0.45 | 1.46 | -1.01 |
Market | -14.21 | 107.31 | 102.00 | 5.31 | -15.30 | -14.51 | -0.79 |
Energy | 33.72 | 2.47 | 4.54 | -2.07 | 0.77 | 1.43 | -0.65 |
AQR-Adage Cluster Residual (Idiosyncratic) Crowding
The stock-specific bets of the AQR-Adage Cluster have grown more crowded as the idiosyncratic volatility of several crowded longs spiked recently. Four stocks account for most of its relative residual risk:
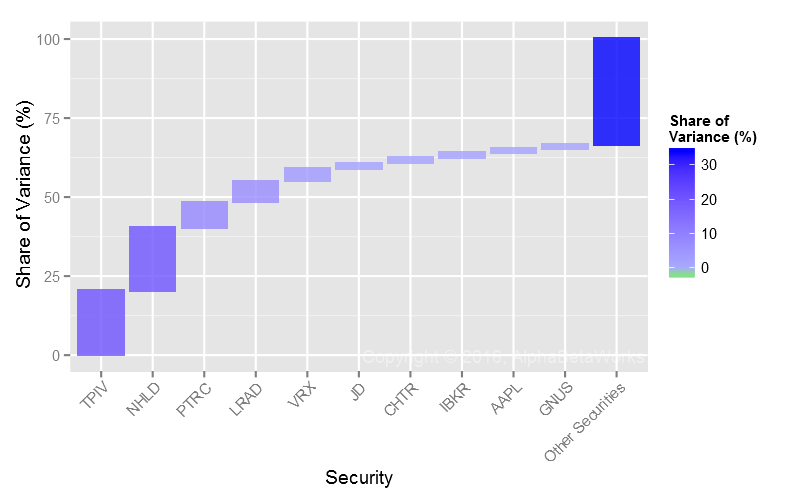
Stocks Contributing Most to Relative Residual Variance of the AQR-Adage Hedge Fund Cluster in Q4 2015
Symbol | Name | Relative Exposure | Residual Volatility | Share of Relative Residual Variance | Share of Relative Total Variance |
TPIV | TapImmune Inc. | 0.41 | 125.70 | 20.11 | 9.21 |
NHLD | National Holdings Corporation | 0.75 | 68.38 | 20.05 | 9.18 |
PTRC | Petro River Oil Corp. | 0.22 | 151.46 | 8.05 | 3.69 |
LRAD | LRAD Corporation | 0.76 | 38.81 | 6.69 | 3.06 |
VRX | Valeant Pharmaceuticals International, Inc. | 0.51 | 43.72 | 3.82 | 1.75 |
JD | JD.com, Inc. Sponsored ADR Class A | 0.49 | 31.91 | 1.86 | 0.85 |
CHTR | Charter Communications, Inc. Class A | 0.75 | 20.31 | 1.76 | 0.81 |
IBKR | Interactive Brokers Group, Inc. Class A | 0.71 | 19.64 | 1.47 | 0.67 |
AAPL | Apple Inc. | -0.81 | 16.25 | 1.33 | 0.61 |
GNUS | Genius Brands International, Inc. | 0.12 | 103.74 | 1.21 | 0.56 |
(Relative exposures and relative variance contribution. All values are in %. Volatility is annualized.)
Idiosyncratic crowding is not the main problem with this cluster, since the expected idiosyncratic tracking error is low (around 1.3%). However, it is vital for fund followers, as it helps explain unexpected volatility in the most crowded names. In fact, several of the crowded names above have shown signs of mass liquidation. It is also worth noting that the crowded names’ from earlier in 2015 presaged subsequent disasters. Valeant Pharmaceuticals (VRX), Micron, Inc. (MU), and Cheniere Natural Gas (LNG) were all featured in our crowding work.
Passivity is a bigger problem still, since allocators to diversified portfolios of hedge funds within this cluster may be paying high fees for a few consensus bets.
Summary
- An analysis of the underlying structure of hedge fund crowding reveals hedge fund clustering – groups of portfolios with similar bets.
- The largest cluster’s factor herding is towards Market (high-beta), short Size (small-cap), and four stock-specific bets (TPIV, NHLD, PTRC, and LRAD).
- Allocators and fund followers unaware of clustering may find themselves in a nearly passive factor portfolio and a handful of consensus stock-specific bets.
The information herein is not represented or warranted to be accurate, correct, complete or timely.
Past performance is no guarantee of future results.
Copyright © 2012-2016, AlphaBetaWorks, a division of Alpha Beta Analytics, LLC. All rights reserved.
Content may not be republished without express written consent.